top
Khám Phá Sự Hòa Quyện Tinh Tế Giữa Chiến Lược Bảo Thủ Và Biến Động: Hướng Đi Đột Phá Cho Hybrid SIM Slot
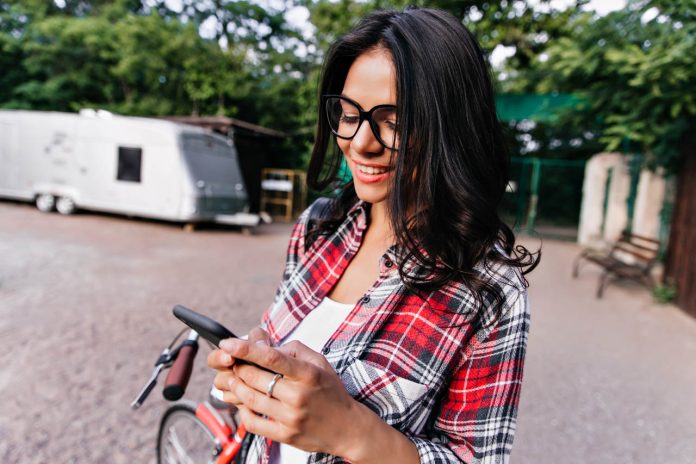
top
Khám Phá Chiến Lược Đầu Tư Đột Phá: Rủi Ro, Mất Mát Ngắn Hạn và Lợi Nhuận Động
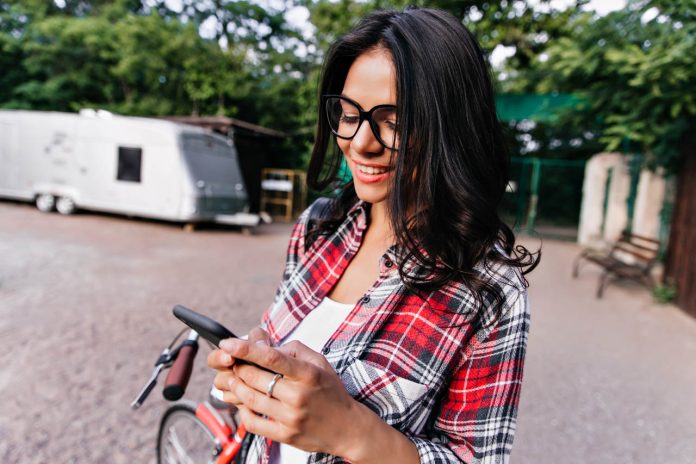
top
绮幻胜机:777老虎机神秘算法全揭秘
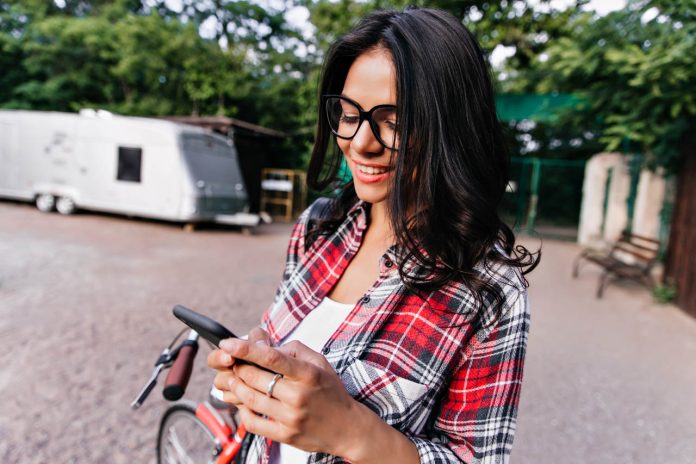
top
Vũ Điệu Đầu Tư: Chiến Lược Slot Booking Hiện Đại và Cơ Hội VIP
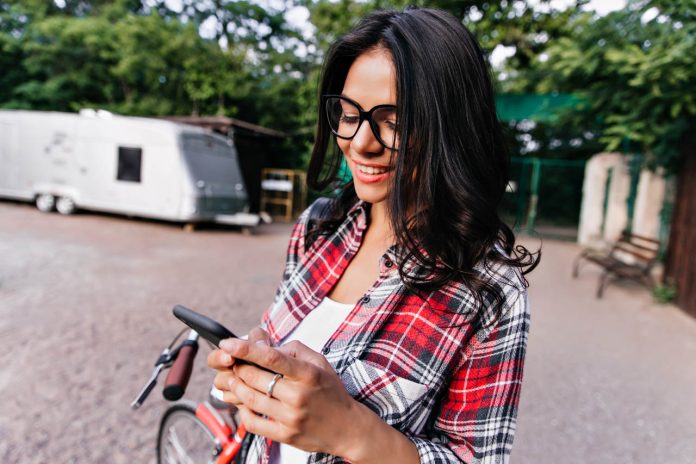
top
Bí ẩn của FreeBonusGames: Từ Flash Đến LowRiskBet – Khi Chiến Lược Giao Thoa Hài Hước
top
Vũ Điệu Chiến Lược: Hòa Quyện May Rủi Và Kỹ Thuật Hiện Đại
top
Sự Bùng Nổ của Game Lớn: Phân Tích Chiến Lược và Tối Ưu Đầu Tư Trò Chơi
top
Cỗ máy tiên phong: Bản chất ẩn chứa của Gametypes và Wageroptimization trong Vũ trụ Game
top
Phá Vỡ Quy Tắc: Hành Trình Khám Phá Thế Giới Slots Winner APK
top
Khám Phá Thế Giới Kỳ Diệu Của Yono Slots: Vận May, Chiến Lược Và Phong Cách
Experience Bliss and Fortune in the Free Casino Mystery Symbols Realm
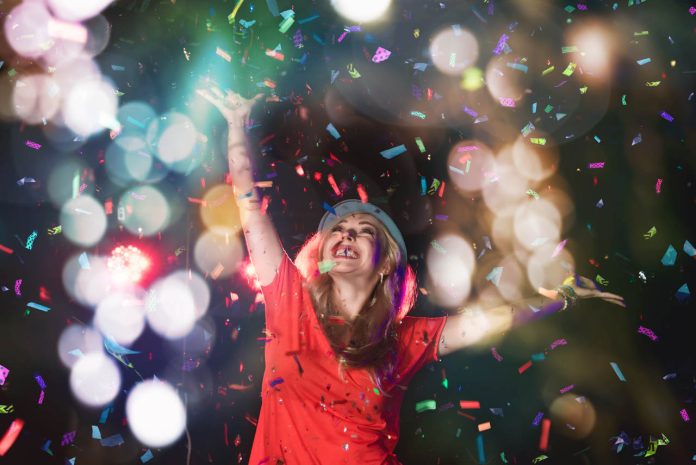
Sáng Tạo Mỗi Vòng Quay: Khi Cơ Hội Gặp Gỡ Tài Năng
Chủ đề
Bước Đột Phá Vô Hạn: Đặt Slot Passport Trong Kỷ Nguyên Rủi Ro & Tăng Trưởng Cân Bằng
Chủ đề
Giai Điệu Số May và Thách Thức Hội Tụ: Casino Slot App 3K Ấn Độ
Chủ đề
Phá Vỡ Giới Hạn: Sự Hòa Quyện của Videopoker và Chiến Lược Cá Độ Mới Mẻ
Chủ đề
Hành Trình Bí Ẩn của Cashround: Những Cơ Hội và Thách Thức Trong Chiến Lược Đầu Tư
Chủ đề
Khám Phá Bí Ẩn Của Slot Win: Phân Tích Chiến Lược và Biểu Tượng Huyền Bí
Chủ đề
Bứt Phá Giới Hạn: Hành Trình Từ Ngân Sách An Toàn đến Ngưỡng Chiến Thắng Đỉnh Cao
Chủ đề
Khám Phá Chiến Lược Roulette: Phân Tích Dữ Liệu Sâu Rộng Mang Lại Niềm Tin và May Mắn
Chủ đề
Ánh Sáng Lấp Lánh: Hòa Quyện Giữa Toán Học Và Rủi Ro Trong Megagame Poker
Chủ đề
Sáng Tạo Đột Phá: Highreel và DualBettingApproach Mở Ra Kỷ Nguyên Cá Cược Mới
Chủ đề
Huyền Bí Của Slot Games Online 3k.top: Bí Quyết Thành Công Độc Đáo
Chủ đề
Step into our free casino realm; wager fearlessly as linear luck guides your thrilling adventure.
Tin tức nóng
Biến Động Thị Trường: Khám Phá Bí Quyết Tối Ưu Khuyến Mãi Trong Ngành Casino
Tin tức nóng
Sự Hòa Quyện Kỳ Diệu của Slot Machine: Chiến Lược Caribbean và Quy Luật Bellcurve
Tin tức nóng
Những Chiến Lược Sáng Tạo Trong Baccarat và Quản Lý Rủi Ro: Hành Trình Vượt Qua Thách Thức
Tin tức nóng
Chinh Phục Những Bí Ẩn của Slot 789: Sự Kết Hợp Giữa Thông Minh và May Mắn
Tin tức nóng
Bùng Nổ Đột Phá: Sự Biến Hữu Hạn của Công Nghệ Casino - Khi newfeatures & bonuswager Thay Đổi Tương Lai
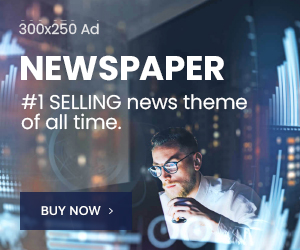
Popular Categories
Embark on a Safe and Thrilling Online Slot Adventure of Positivity
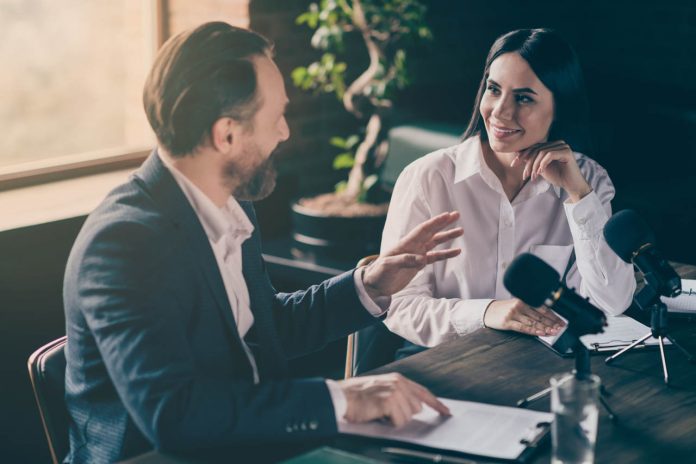
Điều Kỳ Diệu của Ứng Dụng Lucky Slots: Chìa Khóa Thành Công với Real Money 3K Top
Tin tức nổi bật
Khám Phá Bí Mật Thành Công của Lucky Slots AppSự ra đời của Lucky Slots App không chỉ đơn thuần là một bước tiến công nghệ trong lĩnh vực giải trí mà ...
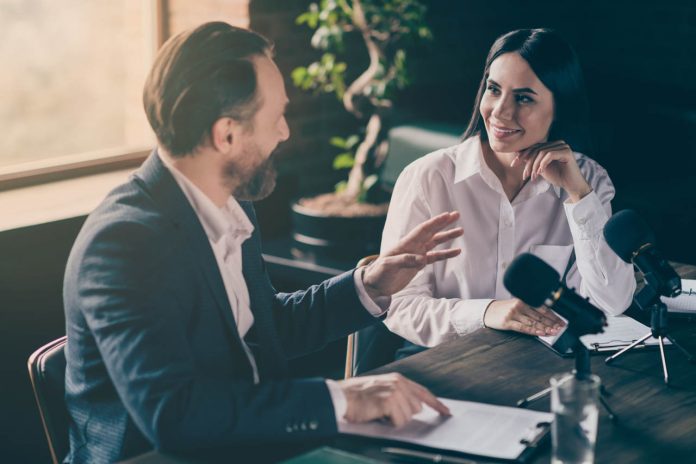
Cơ Chế VIPCashback: Sự Kết Hợp của Chiến Lược Ultrawin và An Toàn Tài Chính
Tin tức nổi bật
Cơ Chế VIPCashback: Sự Kết Hợp của Chiến Lược Ultrawin và An Toàn Tài ChínhTrong bối cảnh thị trường tài chính toàn cầu biến động, việc áp dụng các ch...
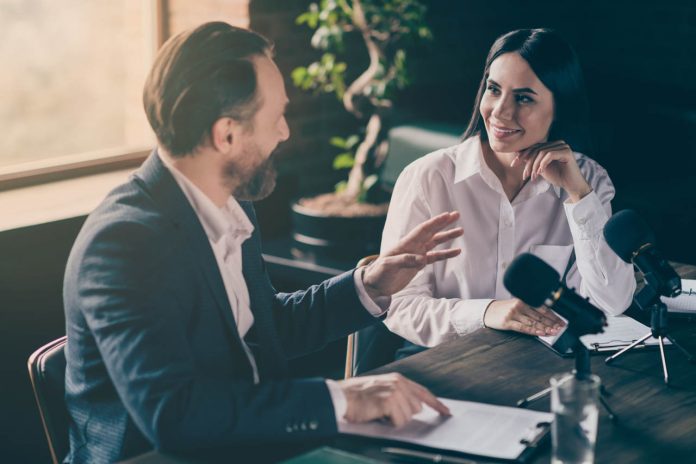
Cuộn Lốc Rực Lửa: Cuộc Phiêu Lưu Bonus, Stochastic và Quản Lý Rủi Ro Trong Cá Cược
Tin tức nổi bật
Cuộn Lốc Rực Lửa: Cuộc Phiêu Lưu Bonus, Stochastic và Quản Lý Rủi Ro Trong Cá CượcBản năng đối đầu với định mệnh đã làm bùng nổ một trải nghiệm cá cượ...
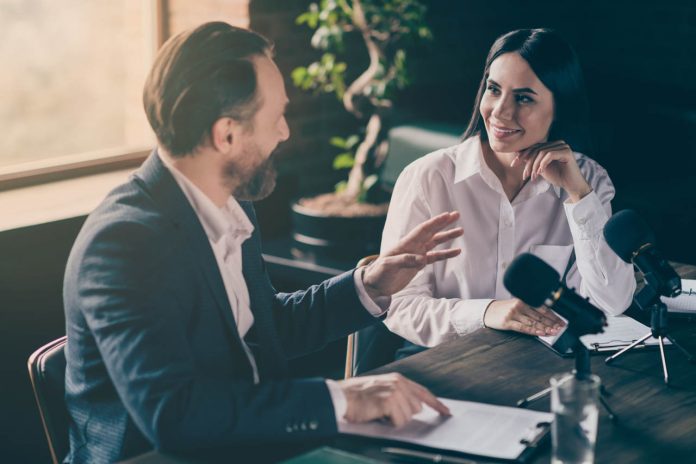
Vượt Qua Giới Hạn Slot Game: Hòa Nhịp giữa Omaha và Chiến Lược Cân Đối Rủi Ro
Tin tức nổi bật
Vượt Qua Giới Hạn Slot Game: Hòa Nhịp giữa Omaha và Chiến Lược Cân Đối Rủi RoPhá vỡ bầu không khí truyền thống, slot game không chỉ là một trò chơi dà...
Experience the thrill of online slots with unpredictable big wins that keep your adrenaline soaring.
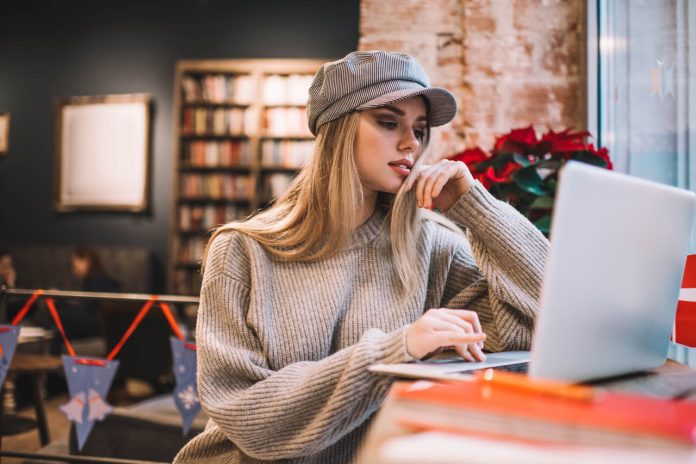
Tiếp Cận Tương Lai: Công Nghệ Lucky Slots và Chiến Lược Cá Cược Góc
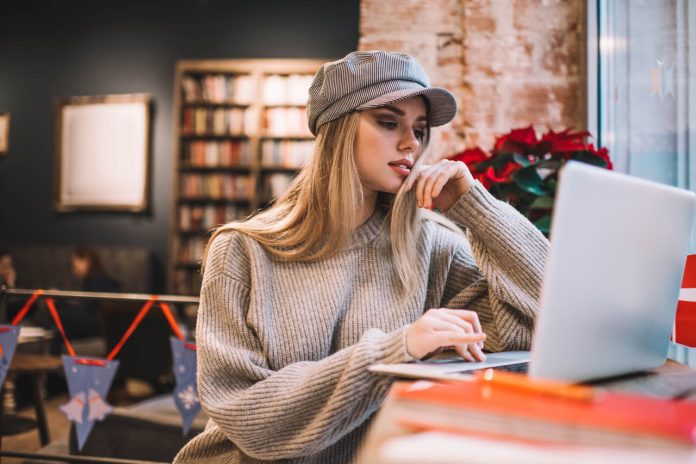
Phân Tích Sâu về Đa Thành Phần Trong Anime: Từ Failurerate đến RandomPlay
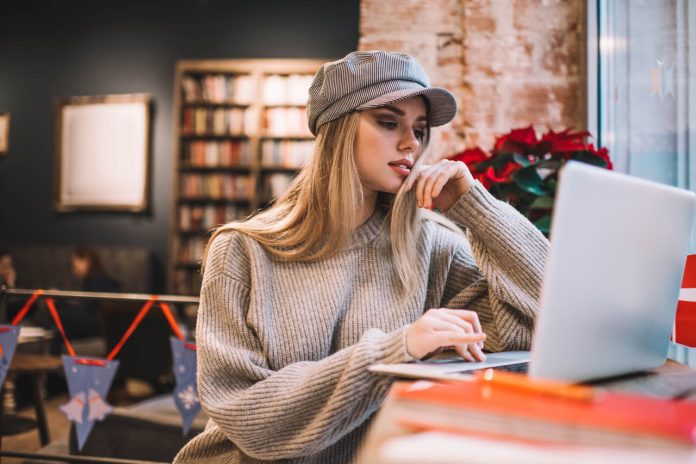
Đột Phá Thế Giới Slot Online: Hòa Mình Vào Cơn Lốc Kịch Tính và Sáng Tạo
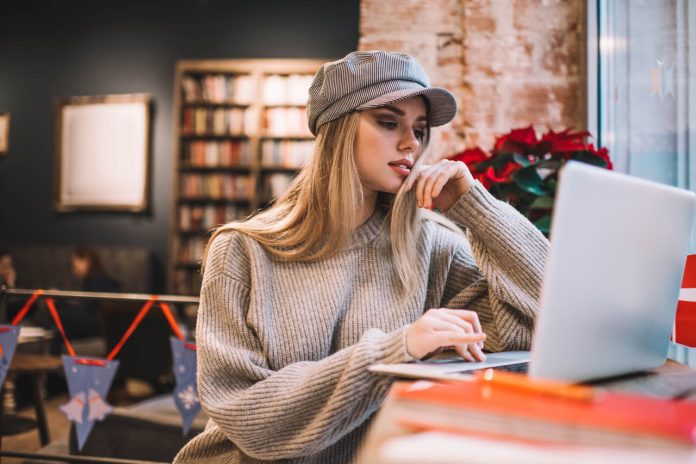
Khai Phá Điểm Mạnh và Thách Thức của My 777 Slots: Giao Thoa Giữa Công Nghệ & Chiến Lược
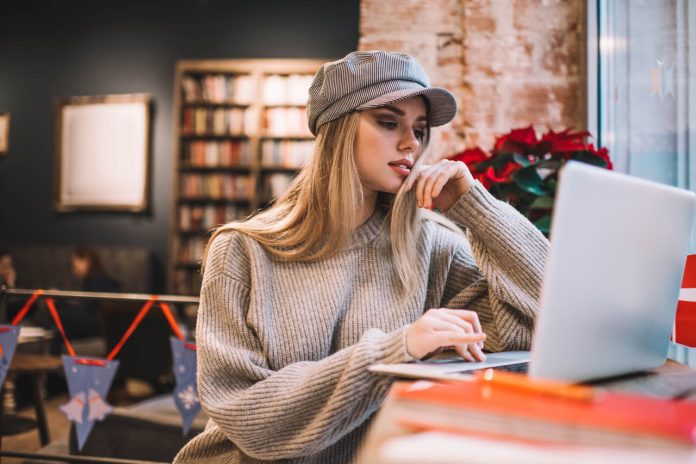
Hành Trình Chạm Vào Tâm Thần May Rủi: Khám Phá Bí Quyết Bonuswin Đầy Hấp Dẫn
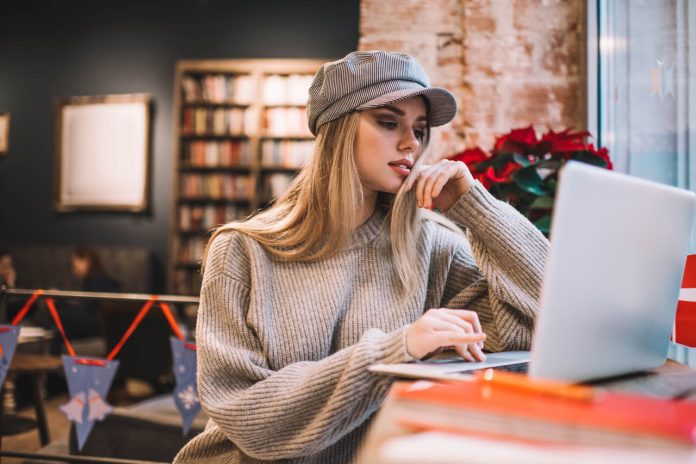
Bùng Nổ Cảm Hứng: Tương Lai Rực Rỡ của Yono Slots 2024
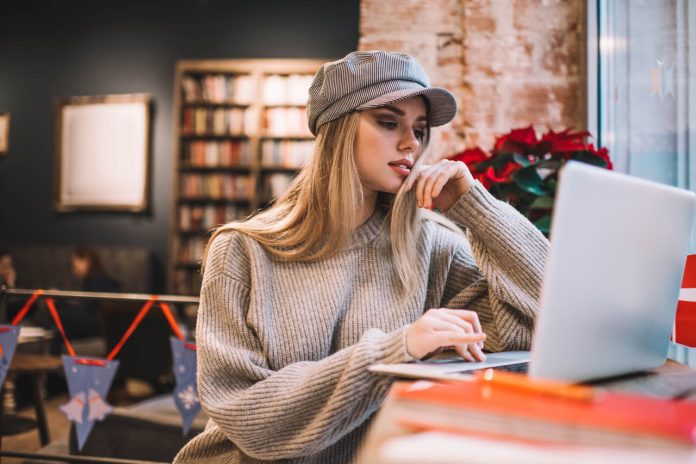
Slot Sôi Động: Cuộc Phiêu Lưu Vào Thế Giới Giải Trí Và Chiến Thắng
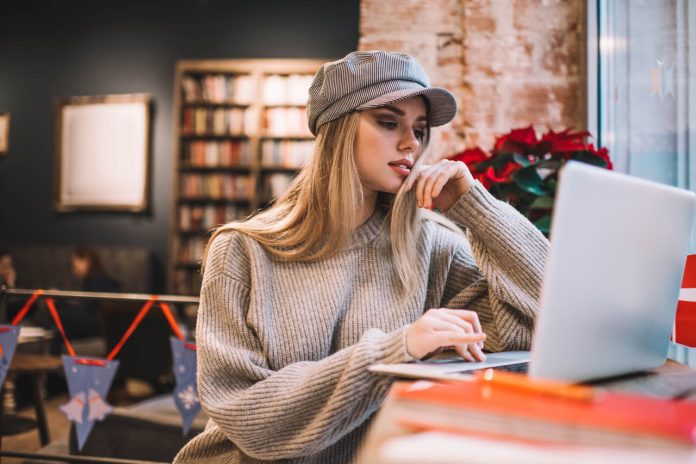
Sự Hòa Tấu Giữa May Rủi và Chiến Lược: Cược An Toàn Trong Thế Giới Bất Ổn
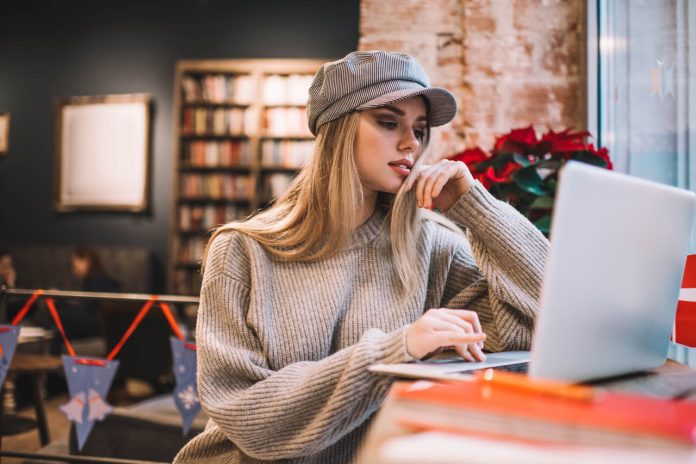
Khám Phá Đa Chiều Ý Nghĩa: Hòa Quyện Sáng Tạo và Trách Nhiệm Trong Wildsymbol
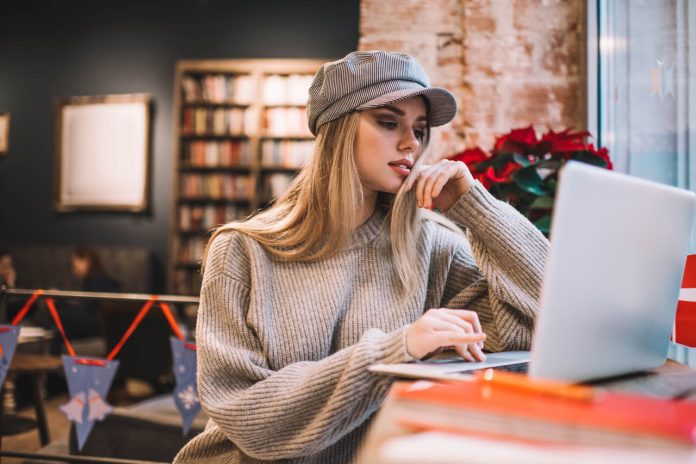
Tiềm Năng Xoay Chuyển: Cân Bằng giữa Dealer và Lợi Nhuận Đa Chiều

Spin, Win, and Embrace Fortune: Claim Your Extra Casino Rewards Now!
Experience thrilling slot action, master betting limits, and seize your chance for colossal casino wins!
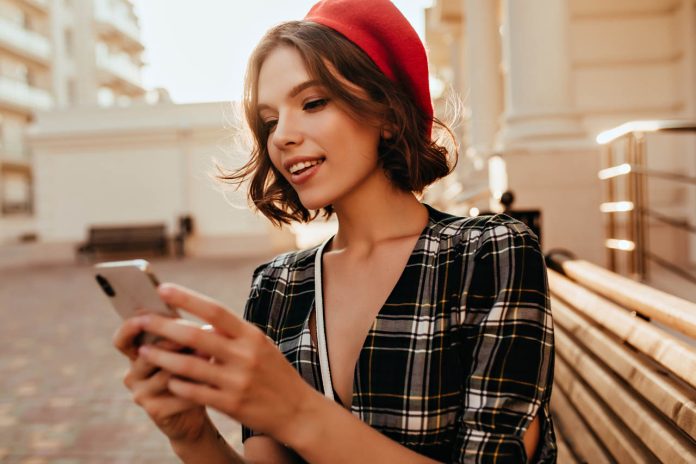
Bùng Nổ Chiến Lược: Khi Poker Và Chương Trình Thưởng Thay Đổi Cuộc Chơi
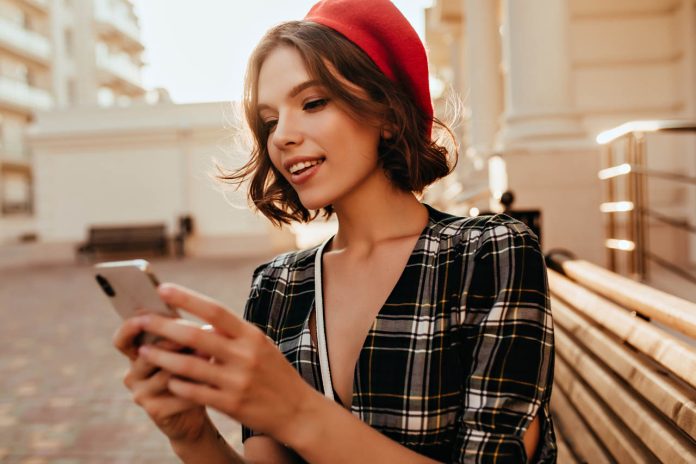
Tương Lai Số Độc Đáo: Hòa Nhập Giữa e Uparjan Slot Booking và Công Nghệ Cá Cược Hiện Đại
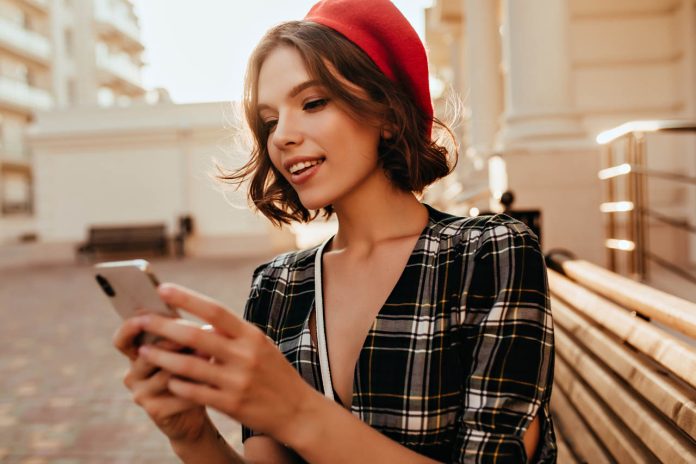
Sức Mạnh Của Chiến Lược: Khám Phá Megaways Và Các Chiến Thuật Cá Cược Tối Ưu
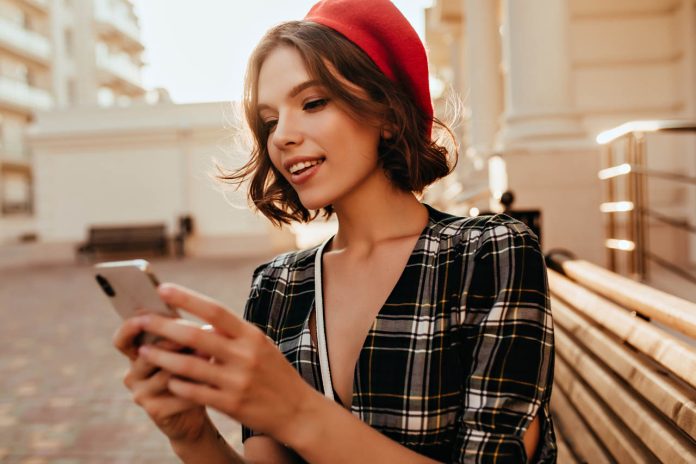
Kỳ Phong Vô Song: Hòa Quyện của Browser, Odds và Chiến Lược DualBankROLL